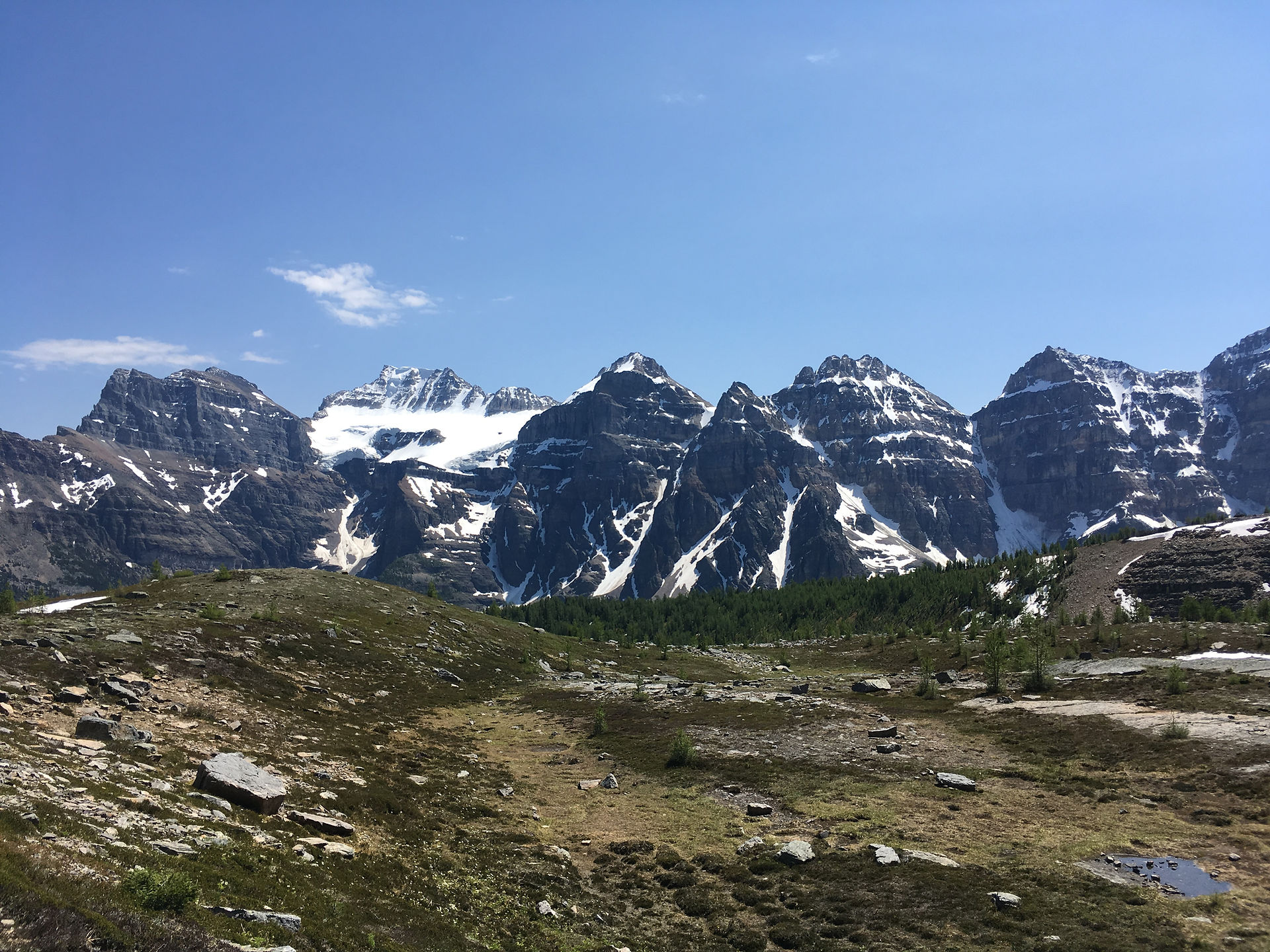

Laboratory experiments have demonstrated a strong, functional correlation between the concentration of eDNA in an environment and organism abundance – on average, abundance can explain approximately 80% of the variation in eDNA concentration observed across experimental replicates (Figure 1). However, when these relationships are extended to natural ecosystems, the relationship between organism abundance and eDNA concentration weakens substantially, explaining between ~50-60% of the variation observed across natural ecosystems (Figure 1).
This is, perhaps, unsurprising – laboratory experiments are highly controlled environments, whereas in natural ecosystems myriad abiotic and biotic factors can impact eDNA production, degradation, and transportation. While laboratory results demonstrate the potential of eDNA as a method to infer abundance, we need to start accounting for ‘eDNA dynamics’ in natural ecosystems and the ecology of its production to further operationalize eDNA as a means to monitor abundance in natural ecosystems. Nevertheless, we concluded that preliminary research is encouraging; with moderate improvements in technology or analytical techniques, eDNA could represent a promising tool for monitoring abundance.
0.73
2
b
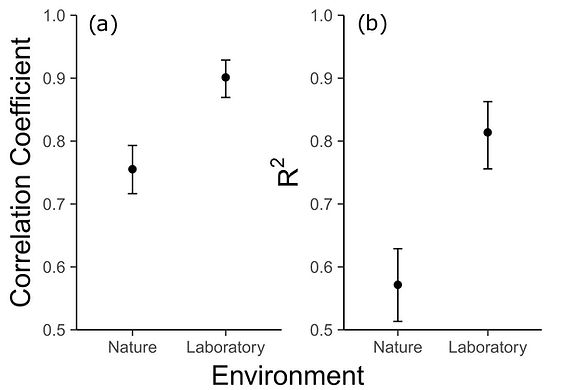
Understanding the ecology of eDNA production is crucial for interpreting quantitative eDNA data. Environmental DNA production is related to both physiological processes (e.g. consumption, excretion) and organism surface area. Physiological models of allometric scaling from the Metabolic Theory of Ecology (Brown et al. 2004, Ecol) are therefore promising approaches to extend to eDNA production. These frameworks assume eDNA production is a function of the mass of an individual organism raised to the power of a scaling coefficient, ‘b’:
eDNA production rate (copies/time) = a * (Individual Mass)
Where the value of the scaling coefficient (‘b’) is <1. In functional terms, this means that although larger individuals will tend to produce more total eDNA, on a per-gram basis (i.e. ‘mass-specific rate’, or copies/gram of mass) they tend to produce less eDNA than smaller individuals. In nine lakes in the Rocky Mountains of Canada, we examined the relationship between brook trout abundance and eDNA. Integrating allometric scaling (e.g. ∑(individual mass )/ha) significantly improved model fit between eDNA concentration and Brook Trout abundance (r = 0.78) relative to traditional metrics (density and biomass, r = 0.59 and 0.63, respectively) (Figure 2).
Figure 1: Mean Pearson Correlation coefficient values and corresponding R between eDNA and density/biomass in natural and laboratory environments. Error bars = SE
2
2
Figure 2: Correlation between weighted mean lake Brook Trout eDNA concentration and three metrics of abundance: (a) density, (b) biomass, and (c) allometrically scaled mass (ASM).
In addition to allometric scaling, metabolic theory and bioenergetics also predicts a strong role of temperature on key physiological rates related to eDNA production. In poikilotherms, physiological rates exhibit a non-linear relationship with temperature – these models could also potentially be extended to eDNA production. Previous published experiments have only evaluated the effect of temperature on eDNA production at two or three temperature points, an insufficient gradient to characterize the shape of the temperature-dependency function of eDNA production (f(T)eDNA) (see Figure 3 for example of f(T) for consumption in a salmonid). Estimating a fine-scale (f(T)eDNA) is a critical step for understanding how eDNA concentrations in an ecosystem fluctuate at both short and long timescales, and could potentially be leveraged to also strengthen correlations between organism abundance and eDNA across natural ecosystems. Quantifying the temperature-dependency function of eDNA production is particularly important for species inhabiting temperate ecosystems.
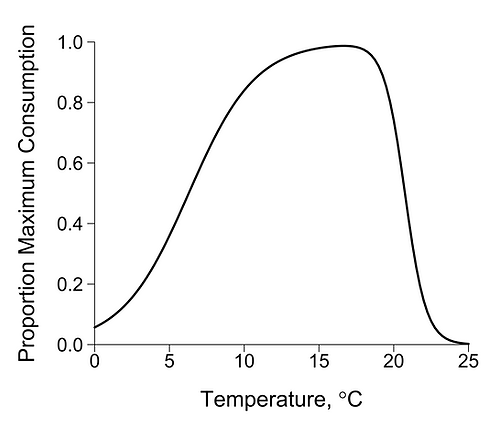
Figure 3: Function describing consumption as a proportion of maximum feeding rate at an ‘optimal’ temperature for Coho salmon (f(T)). Similar functions could be adapted to model eDNA production. Figure adapted from data presented in Stewart and Ibarra 1991.
Building on individual-level frameworks developed from the Metabolic Theory of Ecology, we derived a ‘general framework’ through which quantitative eDNA data can be transformed to simultaneously reflect both population-level N and biomass. Notably, our framework demonstrates that even under circumstances where eDNA is assumed to scale linearly with either numerical abundance or biomass (e.g. the allometric scaling coefficient (‘b’) is equal to 0 or 1, respectively), deriving the other metric necessitates a ‘correction’ when body size variation among populations exists. We then validated our ‘general framework’ using data from two previously published studies: (i) a marine eDNA metabarcoding dataset; and (ii) a freshwater single-species qPCR dataset. Using a Bayesian modeling framework, we estimated the value of the allometric scaling coefficient that jointly optimized the relationship between N, biomass, and ‘corrected’ eDNA data to be 0.82 and 0.77 in Case Studies (i) and (ii), respectively. These estimates closely match expected scaling coefficients estimated in previous work on Teleost fish metabolic rates. We also demonstrate that correcting quantitative eDNA can significantly improve correspondence between eDNA- and traditionally-derived quantitative community biodiversity metrics (e.g., Shannon index and Bray-Curtis dissimilarity) under some circumstances. Collectively, we show that quantitative eDNA data is unlikely to correspond exactly to either N or biomass, but can be ‘corrected’ to reflect both through our unifying joint modelling framework. This framework can also be further expanded to include other variables that might impact eDNA pseudo-steady-state concentrations in natural ecosystems (e.g., temperature, pH, and phenology).
This research demonstrated allometric scaling in eDNA production on an intra-specific basis; I hypothesize that similar general interspecific allometric scaling relationships exist for eDNA production, with important implications for monitoring biodiversity using community-level eDNA approaches (e.g. metabarcoding).
Figure 4: Directed Acyclic Graph demonstrating how raw trawl and eDNA data (squares) are used to estimate a latent parameter (allometric scaling coefficient ‘b’; circle). Mean mass and eDNA data, in combination with the latent ‘b’ parameter, are then used to derive Numerical abundance (N), biomass, and community biodiversity metrics (Shannon Index and Bray-Curtis).
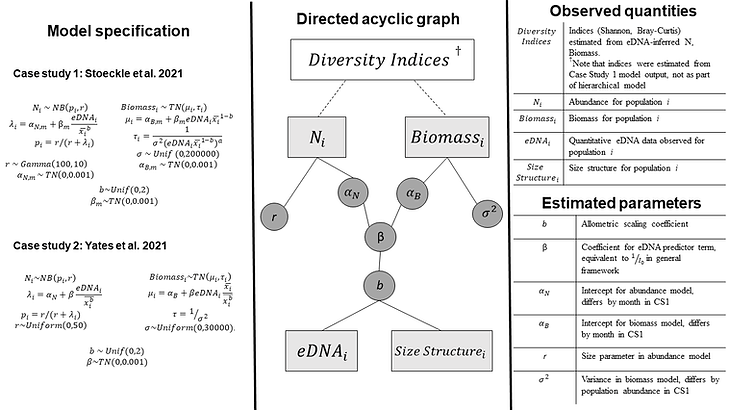
Environmental RNA has long been assumed to be unreliable due the rapidity of its degradation. However, novel research has shown that eRNA can be reliably detected up to twelve hours after its production. Conspecific organisms typically possess near-identical DNA, limiting the resolution of eDNA monitoring applications to species detection. It is not possible, for example, to differentiate eDNA produced by conspecific juveniles and adults. Environmental RNA, on the other hand, reflects actively transcribed genes – and some genes can be ‘turned off’ or ‘turned on’ (or upregulated/downregulated) depending on, for example, an organism’s sex, life-history stage, or environmental conditions. It may therefore be possible to develop eRNA assays targeting gene transcription differences between individuals from the same species (see Yates et al. 2021, TREE) (Figure 4). This could allow researchers to push the resolution of eRNA assays beyond species detection limits to quantify different life-history stages, stress responses to environmental conditions (e.g. heat shock protein expression), or organism ‘health’; this would represent a significant break-through in the use of eRNA for biodiversity monitoring.
I am currently conducting studies on Daphnia and fish examining eRNA to monitor the physiological status of progenitor organisms. I am also conducting trials to examine the effectiveness of different preservation and extraction protocols on the recovery of eRNA.
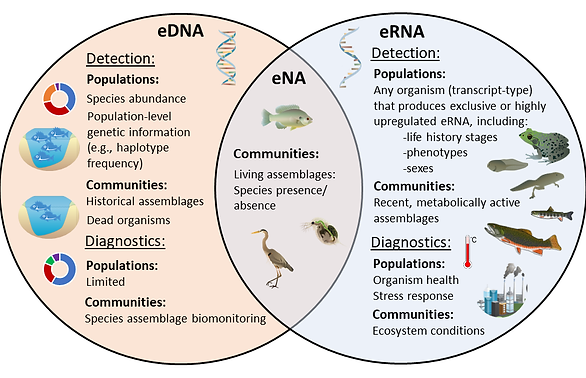
Figure 5: Environmental RNA (eRNA) has the potential to significantly improve population and community biological monitoring applications by moving beyond environmental DNA (eDNA) detection of species presence, abundance, and diversity towards enhanced detection and diagnostics of population and community characteristics.

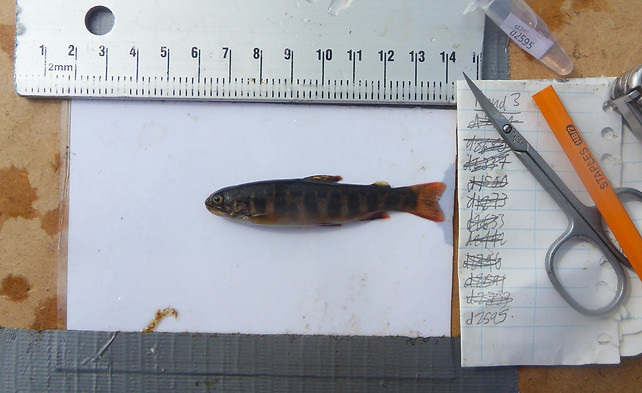
Genetic theory predicts that small population size and low genetic diversity will have negative consequences on both the fitness of individuals within those populations and their capacity to adapt to changing environments. Using meta-analysis, common garden experiments, and field experiments my research has investigated how small populations persist and adapt to novel environmental conditions.
Despite theoretical predictions that large populations should exhibit higher additive genetic variation, several meta-analyses we conducted found little evidence that small populations exhibited reduced heritability in a broad suite of traits (Wood et al. 2016, Evol. Appl.) or exhibited reduced survival or growth (proxies for ‘fitness’) when translocated to novel environments (Yates et al. 2014, Evol. Appl.).
To further investigate these issues we conducted a number of empirical experiments (in both the field and the lab) to examine how small populations of Brook Trout from Cape Race, Newfoundland (Canada) survive and adapt to novel conditions. For my PhD research, I conducted ~100 experimental translocations in which I transplanted juvenile Brook Trout from 12 populations (that varied in census size and genetic diversity) to novel, previously uninhabited ponds that represented a gradient of ecologically important variables (e.g. pH, temperature, etc.) (see Figure 5) and examined their subsequent survival and growth (‘fitness’ proxies). We found no evidence that populations with low genetic diversity or small size exhibited reduced survival or growth in large ponds, which was instead determined largely be the environmental conditions of the ponds themselves or the initial size of individuals at the time of translocation (Figure 6). These findings demonstrate that small populations may be capable of persisting at small population size and low genetic diversity, and also could represent reservoirs of important genetic diversity. Our findings also highlight the importance of preserving suitable habitat for populations threatened by environmental change.
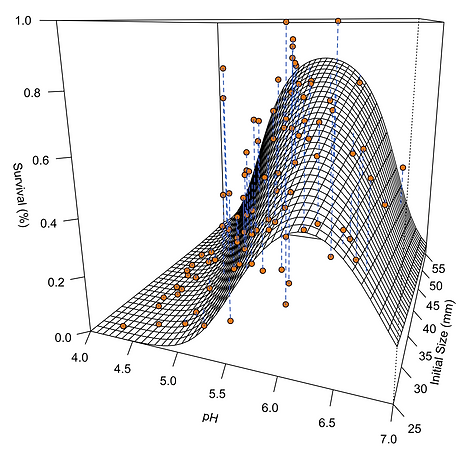
We also examined if translocated individuals exhibited a ‘release of plasticity’ when exposed to novel environmental change. Phenotypic reaction norms are often shaped and constrained by selection and are important for allowing organisms to respond to environmental change. However, selection cannot constrain reaction norms for environmental conditions that populations have not experienced. Consequently, cryptic neutral genetic variation for the reaction norm can accumulate such that a release of phenotypic variation occurs upon exposure to novel conditions. Most genomic diversity behaves as if functionally neutral. Therefore, genome-wide diversity metrics may correlate with levels of cryptic genetic variation and, as a result, exhibit a positive relationship with a release of phenotypic variation in novel environments. We assessed reaction norms for morphological traits (body size and four morphometric relative warps) across pond environmental gradients and evaluated the effect of genome-wide heterozygosity on phenotypic variability. All traits displayed plastic reaction norms. Overall, we found some evidence that a release of phenotypic variation consistent with cryptic genetic variation can occur in novel environmental conditions. However, the extent to which this release correlated with average genome-wide diversity was limited to only one of five traits examined. Our results suggest a limited link between genomic diversity and the accumulation of cryptic genetic variation in reaction norms.
Figure 6: Recapturing Brook Trout transplanted to small, isolated, and previously fishless ponds that exhibited a gradient of ecologically important conditions.
Figure 7: The effect of pH and mean initial size at translocation on survival for translocated brook trout (n = 97 ponds)
Figure 8: Taking a picture of a recaptured translocated Brook Trout for future morphological analyses.
Future research in this system will investigate the potential buffering effect of genome duplication for small populations; Brook Trout are residual polyploids, which may provide some buffering capacity against the potential negative effects of small population size on long-term fitness.